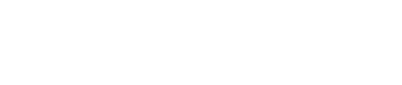
Next-generation data centers and generative AI
Spiros Liolis | June 2023
The global artificial intelligence (AI) market is expected to increase from $11.3 billion in 2023 to $51.8 billion by 2028, at a compound annual growth rate of 35.6% over the course of the forecast period, according to market research company MarketsandMarkets.
Much of this growth can be attributed to generative AI, or systems that can generate new content, like text, images, or sounds, in response to prompts given by users – versus predictive AI, which provides answers or predictions based on existing data.
In fact, there aren’t many conversations that I have today, either business or leisure, that don’t mention Open AI’s ChatGPT, one of the latest advances in the generative AI space. Topics vary from how it’s used to write emails and messages, plan events and vacations, or just for fun.
What's the environmental impact of generative AI?
A recent conversation with industry leaders led me to consider the environmental costs of the generative AI model and its impact on data center facilities. My research started by entering prompts into the resource itself:
How much data center energy in kilowatts (kW) does ChatGPT consume daily to respond to typical user queries? On average, how many queries does ChatGPT respond to?
ChatGPT: As an AI language model, I do not consume energy directly, as I exist solely in computer servers and use the computing power provided by those servers to generate responses to user queries. […] However, some estimates suggest that large data centers can consume anywhere from several megawatts to several hundred megawatts of power, depending on their size and usage. […] However, I can give you a rough estimate based on some assumptions. […] So, a very rough approximation of the energy usage for a single forward pass to answer a typical query is around 0.00085 kilowatt hours (kWh).
Ongoing research on the subject has revealed some surprising elements about energy consumption of the model.
- According to some models, it is estimated that training of GPT-3 consumed 1,287 megawatt-hours (MWh), which emitted 552 tons carbon dioxide equivalent (CO2e)
- The GPT-3 model, which has 175 billion parameters, may require thousands of graphics processing unit (GPU) chips (estimated at 30,000) and millions of GPU-hours to generate a single response
- A data scientist has estimated that in January 2023 alone ChatGPT’s electricity consumption may have been up to 23,364,000 kWh
This is an impressive use of energy from a single generative AI model.
There are many generative AI technologies and models in use today, like DALL-E and GPT-3, among others, and while the technology is still in its infancy, it’s starting to make waves in many industries, including marketing and media, financial services, gaming, 3D, and video, fashion, textiles, and other material sciences, to create novel designs and experiences.
The technology allows engineers, scientists, designers, and artists to quickly experiment with different styles, composites, and materials, resulting in more creative, efficient, environment friendly, and personalized products. Together with digital twin generation and testing, generative AI is projected to be one of the fastest growing markets.
Data centers, the benefactor and beneficiary
The impact of generative AI on data centers will be significant as these systems require large amounts of computational power and storage to train and generate new content, and the basis of generative AI is just that – to analyze massive amounts of historical data to generate new content, which in turn can be used in the future.
As data centers continue to evolve and expand, traditional methods of the data center lifecycle may no longer be sufficient. The level of complexity of the environment requires new thinking. Generative AI is transforming how data centers are designed, operated, optimized, and maintained. The very benefits of generative AI can be used to revolutionize the way data centers are built and managed.
Data centers, therefore, will be both a benefactor to generative AI, as well as a beneficiary.
How data centers are enabling generative AI
Data centers support generative AI through high-performance computing (HPC), hosting specialized hardware, data storage, and networking. One of the largest challenges associated with generative AI is the need for computing intensive resources, such as GPUs, to process the massive amounts of data required to train these systems effectively. This can lead to significant increases in power consumption and cooling requirements for data centers, as well as the need for more advanced infrastructure and networking to handle the increased demand.
Building modern, resilient, HPC-grade data center infrastructure, which is also optimized for generative AI workloads, provides a great opportunity for data center operators to cater to this niche market.
The early adopters of generative AI are players in the generalist areas. Typical use cases include basic or advanced searching and media and image creation based on existing public data from the internet, such as creating papers, campaign emails, and even competitive intelligence pieces.
The early hysteria of generative AI is now giving ground to more tailored, industry specific use cases across industries, including the architectural, construction and engineering (ACE) services sector. These use cases base their generative AI not only on the internet, but perhaps more importantly, on large internal data sets, knowledge libraries, and tenured employees, together with forward-thinking professionals in the organization.
While data center facilities have often been considered warehouse buildings that lack design appeal, today architects and engineers are using generative AI solutions, such as DALL-E and Midjourney, to design conceptual building images for their projects. Drawings and sketches that would take weeks to generate and hours of computing to render, now take a few keystrokes and clicks and can be modified on the spot.
What’s more, generative AI can deliver benefits during the detailed design and construction phases by analyzing design options, consuming criteria as input (i.e., Uptime Institute, IA-942 or other mechanical electrical power (MEP)) and optimizing space power cooling (SPC) outputs. It can also assist with scheduling options, material movement, building machinery like cranes, and running what-if scenarios to check on the scheduling impacts and report on progress versus planned, identify delays in the supply chain, and even recommend recovery or fast-track steps.
Beyond architectural design, data centers are also resource consuming facilities. They consume vast amounts of energy, making resource efficiency a top priority. Generative AI technology has a role to play in the data center infrastructure management (DCIM), operation, and maintenance of the facility. It can model and optimize the entire electromechanical ecosystem (i.e., cooling, airflow, and power distribution) and identify areas for energy savings, asset deployment, and capacity management decisions. It can also identify patterns and event correlations that may indicate equipment failures and allow facility managers to plan maintenance while reducing the risk of downtime.
Leading innovation
While generative AI is a powerful technology for optimizing a number of data center facility aspects, it comes with its own limitations and drawbacks.
First, as with other AI or data driven technologies, generative AI models require a significant amount of high-quality data to be effective. Processing vast amounts of data requires fast access to computing, storage, and network fabrics. Specifically for data center construction, such data may be difficult to obtain due to the complexity and variability of the construction process, as well as the variety of data sources over the years, including legacy infrastructure systems as well as CAD files, scheduling files, and spreadsheets, among others.
With such variety and complexity, generative AI models may not be able to account for all variables and constraints involved in data center construction, which could limit their effectiveness.
Second, current generative AI models may not design solutions that are practical or feasible in the real world, at a given location. For example, a model could generate a design that is optimized for energy efficiency but may not be structurally sound or meet regulatory requirements.
Finally, generative AI is not a replacement for human experience or expertise. While it can help optimize some aspects of data center construction, it should always be used in conjunction with human knowledge, practical experience, and pragmatic judgment to ensure the final product is safe, efficient, and effective.
Ramboll can help
Ramboll is a leader in the data centers industry and builds high-performance computing (HPC)-scale facilities that ensure optimal performance, reliability, and energy efficiency. Designing a data center that will accommodate generative AI workloads requires careful planning and consideration of various factors. We take the necessary steps when designing demanding critical facilities including:
- Assessing requirements of HPC workloads, including the types of applications, size of datasets, level of concurrency, and expected performance
- Ensuring the data center has adequate cooling systems (i.e., air conditioning and/or liquid cooling)
- Ensuring reliable and sufficient power while implementing power management techniques, such as dynamic voltage and frequency scaling (DVFS)
- Selecting energy-efficient MEP infrastructure that can meet the requirements of the HPC workloads
We are leading innovation on the use of generative AI technologies by developing solutions in collaboration with experts in data center construction to assure that years of human expertise, together with leading-edge technology, create data center facilities that are optimized for performance, reliability, and energy efficiency now and well into the future.
*Images in this article were created with the assistance of OpenAI's DALL·E.
Want to know more?
Sign up to receive updates, insights, and perspectives from our experts related to data centers and mission critical facilities.
![]()
|
About the author Spiros Liolis Spiros has built a reputation as a technology visionary and transformation catalyst. With over 20 years of experience, Spiros is known for maximizing revenue and growth by driving forward-thinking business development strategies, revolutionary solution development, and proactive sales enablement. His extensive background spanning data centers, digital transformation, emerging technology innovation, and cyber resilience enables him to provide data center strategy analysis to help clients address their forward mission goals – including IT and network architecture, application portfolios, data center sourcing needs, topology, reliability, and associated financial analysis for decision support requirements. Spiros holds multiple technical certifications and is a member of the distinguished Forbes Technology Council. |